How problematic is AI technology?
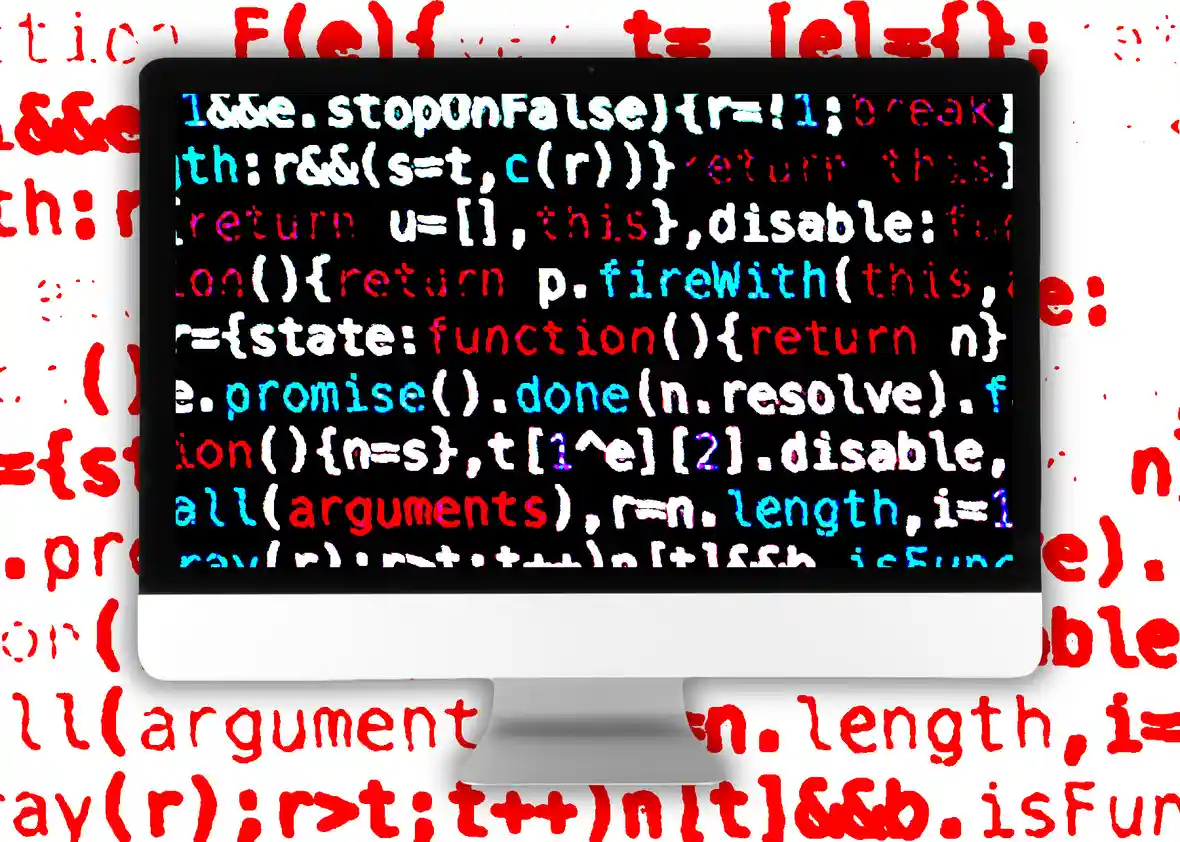
Getting to the crux is writer Matt Burgess, whose new book Artificial Intelligence – part of the WIRED series – weighs up the pros and cons of surveillance, personal data and face recognition.
Society
Words: Matt Burgess
Between 2015 and 2020 people applying for visas to enter the United Kingdom to work, study or visit loved ones would fill in the paperwork in the usual way, and that data would then be handed over to an algorithm to assess.
It would give them a rating: red, amber or green. Of those being assessed as green 96.3 per cent were waved through. Those marked as red – the ‘riskiest’ category – weren’t automatically rejected, but were subject to further checks, with senior staff being brought in to check the data and make a final decision. This partially automated process, run by the Home Office, ultimately approved 48 per cent of red applications. Those using it trusted its decisions.
But there was a problem. Although the intention behind the system was laudable – to make visa applications faster, more efficient and less bureaucratic – the underlying technology, known as the streaming tool, was flawed. It was plagued with data, transparency and process problems that resulted in unfair decisions. In particular, it judged people to be high-risk on the basis of their country of origin rather than on carefully considered personal criteria. ‘They kept this list of undesirable nations where simply by making an application coming from a particular country – and they refused to give us the name of the countries – that person would be more likely to be streamed amber or red,’ says Cori Crider, the founder of legal group Foxglove.
In the spring of 2020 Foxglove challenged the algorithmic process, arguing that it broke equality and data protection laws. Days before the case was due to reach court the UK government scrapped the tool, admitting it needed to scrutinise ‘issues around unconscious bias and the use of nationality generally in the streaming tool’.
However, it rejected the suggestions that the system was breaking any laws. Months later, in the midst of the coronavirus pandemic, a similar algorithm attempted to predict English students’ A‑Level exam grades. It, too, used historical data to make decisions about individuals’ futures. It, too, was flawed. Widespread protest ensued, with students taking to the streets and chanting ‘Fuck the algorithm’.
Neither system involved particularly complex algorithms – and neither used artificial intelligence. But what they both demonstrate is the inherently risky nature of data. Whatever we might be tempted to assume, it is rarely, if ever, neutral. Since it is ultimately created by humans, it captures our prejudices. The visa system was founded on data that made biased assumptions about people’s countries of origin. The exams system predicted results based in part on the previous track record of individual pupils’ schools. Both are warnings for the future.
Since data lies at the heart of AI, it follows that AI is not free from prejudice. Bad data put into a system results in bad data outputs. There are three common forms of bias – although research has identified more than 20 types of bias, plus other types of discrimination and unfairness that can be present in AI setups.
With latent bias, an algorithm correlates its results with such characteristics in the data as gender, race, income and more, so potentially perpetuating historical forms of bias: for example, a system may ‘learn’ that doctors are male because the historical data it has been trained on shows doctors as being male. (Amazon had to scrap an AI hiring tool it was using, which was trained upon ten years of CV data, as the system surmised that since men had historically been hired more often than women, they must be better.)
With selection bias results are distorted by a dataset that over-represents one group of people. (An AI created to judge a beauty contest selected mostly white winners, as the dataset it was trained upon mostly contained images of white people.) With interaction bias a system learns from the prejudices people display when they interact with it. (Microsoft’s chatbot, Tay, which was launched on Twitter in 2016, became more coherent within its first twenty-four hours of use, but also repeated all the sexist and racist language people had sent its way.)
All these biases show the risks of using data to predict future outcomes. And within AI the problem has been pronounced. Take law enforcement, for example. In the US in particular the police have come to use predictive systems when they’re seeking to assess how likely someone is to re-offend, or whether they should be granted bail, or when spikes in crimes are likely to happen.
The trouble is that such systems inevitably target individuals and communities that have historically been the focus of particular police attention. ‘If you’re using problematic data, you’re going to get problematic policing,’ explains Renée Cummings, a researcher specialising in AI in the criminal justice sector and its discriminatory effects. In the US people who are black are more than twice as likely to be arrested as white people. They are more likely to be stopped without cause by police, and black men are 2.5 times more likely to be killed by police than white men. ‘It starts from a place of bias,’ Cummings says. ‘AI has really amplified the biases and the discrimination that has been a part of the system.’
A 2016 investigation by ProPublica revealed how predictive software, called COMPAS, was biased against black people. A further study of the tool, which can determine risk, found that it is ‘not well calibrated for Hispanics.’ It was said to over-predict risk scores for Hispanic people and was marked by an inability to make accurate predictions. AI can also perpetuate police misdemeanours. A study by researchers at New York University’s AI Now Institute of thirteen US jurisdictions where predictive policing tools have been in operation concluded that ‘illegal police practices can significantly distort the data that is collected, and the risks that dirty data will still be used for law enforcement and other purposes.
Even when race data is stripped from predictive policing AI systems (a frequent requirement of equalities laws), problems remain. Rashida Richardson, the author of the AI Now paper, who is now a visiting scholar at Rutgers Law School, points out that there are numerous forms of data that can serve as proxies for race. Location is one. If you live in an area that is already heavily policed because of its racial composition, it follows that there will be more police reports on file and so a great likelihood of AI deeming your area to be crime-ridden.
Other data profiles that can lead to distortion include age and social links. One predictive policing tool used in the Netherlands employs demographic data such as the number of one-parent households, the number of people receiving social benefits, and the number of non-Western immigrants as factors in determining how likely a crime is to happen in a particular area. The problem with all this is that while the data collected may sometimes contain useful pointers, it is not neutrally predictive. ‘Police data is more likely to reflect the practices, policies and priorities of a police department and environment of policing than necessarily crime trends,’ Richardson explains.
She questions whether predictive policing technologies are a reflection of what happens in communities: ‘The reality is, if it’s relying mostly on police data, which often is not collected for the subsequent use for some type of data analysis, then it’s always going to have some type of permanent flaw in it that makes it hard to use for any purpose in policing.’ There is currently little evidence to demonstrate that predictive or AI risk assessment tools actually work. Studies showing that algorithmic decision tools can make better predictions than humans are few in number, and because police forces are generally tight-lipped about their use of AI technologies their data has not often been independently analysed and verified.
However, one Los Angeles Police Department review of a system called PredPol, which is used in multiple places across the US, said it was ‘difficult to draw conclusions about the effectiveness of the system in reducing vehicle or other crime’. In June 2020, more than 1,400 mathematicians signed an open letter stating that they did not believe the field should be collaborating with police on these systems.
It also demanded that audits be introduced for those systems already in place. ‘It is simply too easy to create a scientific veneer for racism,’ the researchers wrote. Cori Crider, who led the legal challenges to flawed statistical systems in the UK, adds that these types of technologies often seem to be used against groups who may not have the means to challenge them. ‘It feels like a lot of the systems are directed at the management and mass management of people who have much less power, social capital, money, all of the rest of it,’ she says. ‘I think that there is a really worrying trend, in that algorithmic management is a way to contain and surveil poor people of colour.’
It’s not just policing where AI bias can rear its head. Housing, employment and financial matters have all suffered from bias problems. Healthcare is emerging as the newest sector to suffer with issues. In the US, for instance, researchers from the University of California, Berkeley, discovered that an algorithm used by insurers and hospitals to manage the care of around 200 million people a year gave lower risk scores to people who self-identified as black than to white people who were equally sick. This was because it determined people’s health scores in part according to how much they had spent on healthcare in a year, the assumption being that sicker people spend more than healthy ones.
However, the data also showed there was $1,800 less per year of care given to black patients than to white patients with the same number of chronic health problems. ‘Less money is spent on Black patients who have the same level of need, and the algorithm thus falsely concludes that Black patients are healthier than equally sick White patients,’ the authors of the study wrote. As a result black people were less likely to be referred for treatments involving more specialist care. Had it been a level playing field, 46.5 per cent of the black patients involved would have been referred. As it was, the percentage stood at 17.7 per cent.
As AI becomes more sophisticated, researchers worry that the connections made by algorithms will become more obscure. Proxies for certain types of data will become harder to identify, and machines may make links between certain types of information that humans don’t associate together, or can’t see because of the scale of the information being crunched. The first signs of this happening are already evident.
In March 2019, for example, the US Department of Housing and Urban Development (HUD) charged Facebook with discrimination over how its targeted advertising for housing works. US advertising laws prohibit discrimination against people based on their colour, race, national origin, sex, religion, disability and family status, and, while Facebook’s system wasn’t explicitly guilty of such discrimination, it emerged that the interests people had, as expressed online, led to their exclusion from particular ads.
‘An algorithm is grouping people not based necessarily on sexual orientation or their skin colour,’ says Sandra Watcher, associate professor and senior research fellow in the law and ethics of AI at the Oxford Internet Institute. She says algorithms group people with similar behaviours. ‘These similarities could be that they all have green shoes, or similarities could be that they eat Indian food on a Tuesday, or that they play video games.’ The problem is that such correlations can lead to wholly false inferences – an algorithm might, for example, conclude that people who wear green shoes do, or don’t, have a tendency to repay their loans on time.
To any human, such a conclusion would be preposterous. To an AI it may seem entirely logical. ‘Even if a bank can explain which data and variables have been used to make a decision (e.g. banking records, income, postcode), the decision turns on inferences drawn from these sources; for example, that the applicant is not a reliable borrower,’ Watcher writes. ‘This is an assumption or prediction about future behaviour that cannot be verified or refuted at the time of decision-making.’
She argues that data protection laws need to evolve to handle the issues that arise when machines make false deductions about us. Organisations using such systems should have to prove the connections they are making are reasonable: they should say why certain data is relevant, why the inferences matter to the decision that’s being made and whether the process is accurate and reliable. If not, Watcher says, more people will suffer at the hands of AIs issuing unfair decisions.
Artificial Intelligence by Matt Burgess is part of the WIRED series published by Penguin Random House and is available to purchase here
